Real-time crash prediction in an urban expressway using disaggregated data
Artículo
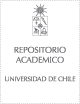
Open/ Download
Publication date
2018Metadata
Show full item record
Cómo citar
Basso, Franco
Cómo citar
Real-time crash prediction in an urban expressway using disaggregated data
Author
Abstract
We develop accident prediction models for a stretch of the urban expressway Autopista Central in
Santiago, Chile, using disaggregate data captured by free-flow toll gates with Automatic Vehicle
Identification (AVI) which, besides their low failure rate, have the advantage of providing disaggregated data per type of vehicle. The process includes a random forest procedure to identify
the strongest precursors of accidents, and the calibration/estimation of two classification models,
namely, Support Vector Machine and Logistic regression. We find that, for this stretch of the
highway, vehicle composition does not play a first-order role. Our best model accurately predicts
67.89% of the accidents with a low false positive rate of 20.94%. These results are among the best
in the literature even though, and as opposed to previous efforts, (i) we do not use only one
partition of the data set for calibration and validation but conduct 300 repetitions of randomly
selected partitions; (ii) our models are validated on the original unbalanced data set (where
accidents are quite rare events), rather than on artificially balanced data.
Indexation
Artículo de publicación SCOPUS
Identifier
URI: https://repositorio.uchile.cl/handle/2250/169356
DOI: 10.1016/j.trc.2017.11.014
ISSN: 0968090X
Quote Item
Transportation Research Part C: Emerging Technologies, Volumen 86, 2018, Pages 202-219
Collections