Uncertainty modeling and spatial prediction by multi-Gaussian kriging: Accounting for an unknown mean value
Artículo
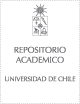
Open/ Download
Publication date
2008-11Metadata
Show full item record
Cómo citar
Emery, Xavier
Cómo citar
Uncertainty modeling and spatial prediction by multi-Gaussian kriging: Accounting for an unknown mean value
Author
Abstract
In the analysis of spatial data, one is often interested in modeling conditional probability distributions, in order to assess the uncertainty in the values of the attribute under study and to predict functions of this attribute.
This work examines three geostatistical models in which the attribute is assumed to be, up to a monotonic transformation, a realization of a Gaussian random field. In the first model, the mean of the Gaussian field is a known parameter and the conditional distributions at any set of locations are Gaussian, with expected values equal to simple kriging predictions and covariance matrix equal to that of the prediction errors. In the second model, the mean value is replaced by a random variable adding to the Gaussian field and whose prior variance is infinitely large, indicating a total lack of prior knowledge on the true mean. It is shown that the conditional distributions are still Gaussian, with expected values equal to ordinary kriging predictions and covariance matrix equal to that of the corresponding prediction errors. The third model considers a random drift that adds to the Gaussian field; the conditional distributions are then obtained by substituting universal kriging for simple or ordinary kriging.
A computer program is provided to calculate recovery functions (tonnages, metal contents and mean values above given thresholds) and uncertainty measures (probability intervals and conditional variances) defined at point or block supports. The concepts are illustrated with a case study consisting of evaluating the recoverable resources in a porphyry copper deposit.
Identifier
URI: https://repositorio.uchile.cl/handle/2250/125159
DOI: 10.1016/j.cageo.2007.12.011
ISSN: 0098-3004
Quote Item
COMPUTERS & GEOSCIENCES Volume: 34 Issue: 11 Pages: 1431-1442 Published: NOV 2008
Collections