A model updating strategy for predicting time series with seasonal patterns
Artículo
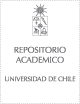
Open/ Download
Publication date
2010Metadata
Show full item record
Cómo citar
Guajardo, José A.
Cómo citar
A model updating strategy for predicting time series with seasonal patterns
Author
Abstract
Traditional methodologies for time series prediction take the series to be predicted and split it into Q1
training, validation, and test sets. The first one serves to construct forecasting models, the second set for
model selection, and the third one is used to evaluate the final model. Different time series approaches
such as ARIMA and exponential smoothing, as well as regression techniques such as neural networks and
support vector regression, have been successfully used to develop forecasting models. A problem that
has not yet received proper attention, however, is how to update such forecastingmodels when new data
arrives, i.e. when a new event of the considered time series occurs.
This paper presents a strategy to update support vector regression based forecasting models for time
series with seasonal patterns. The basic idea of this updating strategy is to add the most recent data to the
training set every time a predefined number of observations takes place. This way, information in new
data is taken into account in model construction. The proposed strategy outperforms the respective
static version in almost all time series studied in this work, considering three different error measures.
Patrocinador
Support by the Scientific Millennium Institute ‘‘Complex
Engineering Systems’’ (www.sistemasdeingenieria.cl) and the
Chilean Fondecyt project 1040926 is greatly acknowledged.
Identifier
URI: https://repositorio.uchile.cl/handle/2250/125416
DOI: doi:10.1016/j.asoc.2009.07.005
Quote Item
Applied Soft Computing 10 (2010) 276–283
Collections