Continuous Modeling of Metabolic Networks With Gene Regulation in Yeast and In Vivo Determination of Rate Parameters
Artículo
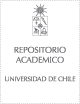
Open/ Download
Publication date
2012-09Metadata
Show full item record
Cómo citar
Moisset, P.
Cómo citar
Continuous Modeling of Metabolic Networks With Gene Regulation in Yeast and In Vivo Determination of Rate Parameters
Author
Abstract
A continuous model of a metabolic network
including gene regulation to simulate metabolic fluxes during
batch cultivation of yeast Saccharomyces cerevisiae was
developed. The metabolic network includes reactions of
glycolysis, gluconeogenesis, glycerol and ethanol synthesis
and consumption, the tricarboxylic acid cycle, and protein
synthesis. Carbon sources considered were glucose and then
ethanol synthesized during growth on glucose. The metabolic
network has 39 fluxes, which represent the action of 50
enzymes and 64 genes and it is coupled with a gene regulation
network which defines enzyme synthesis (activities) and
incorporates regulation by glucose (enzyme induction and
repression), modeled using ordinary differential equations.
The model includes enzyme kinetics, equations that follow
both mass-action law and transport as well as inducible,
repressible, and constitutive enzymes of metabolism. The
model was able to simulate a fermentation of S. cerevisiae
during the exponential growth phase on glucose and the
exponential growth phase on ethanol using only one set of
kinetic parameters. All fluxes in the continuous model
followed the behavior shown by the metabolic flux analysis
(MFA) obtained from experimental results. The differences
obtained between the fluxes given by the model and the
fluxes determined by the MFA do not exceed 25% in 75% of
the cases during exponential growth on glucose, and 20% in
90% of the cases during exponential growth on ethanol.
Furthermore, the adjustment of the fermentation profiles of
biomass, glucose, and ethanol were 95%, 95%, and 79%,
respectively. With these results the simulation was considered
successful. A comparison between the simulation of the
continuous model and the experimental data of the diauxic
yeast fermentation for glucose, biomass, and ethanol, shows
an extremely good match using the parameters found. The
small discrepancies between the fluxes obtained through
MFA and those predicted by the differential equations, as
well as the good match between the profiles of glucose,
biomass, and ethanol, and our simulation, show that this
simple model, that does not rely on complex kinetic expressions,
is able to capture the global behavior of the experimental
data. Also, the determination of parameters using a
straightforward minimization technique using data at only
two points in time was sufficient to produce a relatively
accurate model. Thus, even with a small amount of experimental
data (rates and not concentrations) it was possible to
estimate the parameters minimizing a simple objective
function. The method proposed allows the obtention of
reasonable parameters and concentrations in a system with a
much larger number of unknowns than equations. Hence a
contribution of this study is to present a convenient way to
find in vivo rate parameters to model metabolic and genetic
networks under different conditions.
General note
Artículo de publicación ISI
Quote Item
Biotechnology and Bioengineering, Vol. 109, No. 9, September, 2012
Collections