Feature selection for Support Vector Machines via Mixed Integer Linear Programming
Artículo
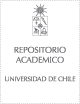
Publication date
2014Metadata
Show full item record
Cómo citar
Maldonado, Sebastián
Cómo citar
Feature selection for Support Vector Machines via Mixed Integer Linear Programming
Abstract
The performance of classification methods, such as Support Vector Machines, depends
heavily on the proper choice of the feature set used to construct the classifier. Feature
selection is an NP-hard problem that has been studied extensively in the literature. Most
strategies propose the elimination of features independently of classifier construction by
exploiting statistical properties of each of the variables, or via greedy search. All such strategies
are heuristic by nature. In this work we propose two different Mixed Integer Linear
Programming formulations based on extensions of Support Vector Machines to overcome
these shortcomings. The proposed approaches perform variable selection simultaneously
with classifier construction using optimization models. We ran experiments on real-world
benchmark datasets, comparing our approaches with well-known feature selection techniques
and obtained better predictions with consistently fewer relevant features.
General note
Artículo de publicación ISI
Patrocinador
Support from the Institute of Complex Engineering Systems (ICM: P-05-004-F, CONICYT: FBO16)
Identifier
URI: https://repositorio.uchile.cl/handle/2250/126859
DOI: DOI: 10.1016/j.ins.2014.03.110
Quote Item
Information Sciences 279 (2014) 163–175
Collections