Particle-Filtering-Based Discharge Time Prognosis for Lithium-Ion Batteries With a Statistical Characterization of Use Profiles
Artículo
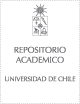
Publication date
2015Metadata
Show full item record
Cómo citar
Pola Contreras, Daniel
Cómo citar
Particle-Filtering-Based Discharge Time Prognosis for Lithium-Ion Batteries With a Statistical Characterization of Use Profiles
Author
Abstract
We present the implementation of a particle-filteringbased
prognostic framework that utilizes statistical characterization
of use profiles to (i) estimate the state-of-charge (SOC), and (ii)
predict the discharge time of energy storage devices (lithium-ion
batteries). The proposed approach uses a novel empirical statespace
model, inspired by battery phenomenology, and particle-filtering
algorithms to estimate SOC and other unknown model parameters
in real-time. The adaptation mechanism used during the
filtering stage improves the convergence of the state estimate, and
provides adequate initial conditions for the prognosis stage. SOC
prognosis is implemented using a particle-filtering-based framework
that considers a statistical characterization of uncertainty for
future discharge profiles based on maximum likelihood estimates
of transition probabilities for a two-state Markov chain. All algorithms
have been trained and validated using experimental data
acquired from one Li-Ion 26650 and two Li-Ion 18650 cells, and
considering different operating conditions.
General note
Artículo de publicación ISI
Patrocinador
Project FONDECYT 1140774
Identifier
URI: https://repositorio.uchile.cl/handle/2250/133035
DOI: DOI: 10.1109/TR.2014.2385069
ISSN: 0018-9529
Quote Item
IEEE Transactions on Reliability, vol. 64, no. 2, June 2015
Collections
The following license files are associated with this item: