A prognostics approach based on the evolution of damage precursors using dynamic Bayesian networks
Artículo
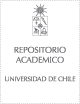
Open/ Download
Publication date
2016Metadata
Show full item record
Cómo citar
Rabiei, Elaheh
Cómo citar
A prognostics approach based on the evolution of damage precursors using dynamic Bayesian networks
Abstract
During the lifetime of a component, microstructural changes emerge at its material level and evolve through time.
Classical empirical degradation models (e.g. Paris Law in fatigue crack growth) are usually established based on monitoring
and estimating well-known direct damage indicators such as crack size. However, by the time the usual inspection
techniques efficiently identify such damage indicators, most of the life of the component would have been expended, and
usually it would be too late to save the component. Therefore, it is important to detect damage at the earliest possible
time. This article presents a new structural health monitoring and damage prognostics framework based on evolution of
damage precursors representing the indirect damage indicators, when conventional direct damage indicator, such as a
crack, is unobservable, inaccessible, or difficult to measure. Dynamic Bayesian network is employed to represent all the
related variables as well as their causal or correlation relationships. Since the degradation model based on damage precursor
evolution is not fully recognized, the methodology needs to be capable of online-learning the degradation process
as well as estimating the damage state. Therefore, the joint particle filtering technique is implemented as an inference
method inside the dynamic Bayesian network to assess both model parameters and damage states simultaneously. The
proposed framework allows the integration of any related sources of information in order to reduce the inherent uncertainties.
Incorporating different types of evidences in dynamic Bayesian network entails advance techniques to identify
and formulate the possible interaction between potentially nonhomogenous variables. This article uses the support vector
regression in order to define generally unknown nonparametric and nonlinear correlation between the input variables.
The methodology is successfully applied to damage estimation and prediction of crack initiation in a metallic alloy
under fatigue. The proposed framework is intended to be general and comprehensive so that it can be implemented in
different applications.
Patrocinador
Chilean National Fund for Scientific and Technological Development (Fondecyt)
1160494
Indexation
Artículo de publicación ISI
Quote Item
Advances in Mechanical Engineering 2016, Vol. 8(9) 1–19
Collections
The following license files are associated with this item: