Multiple classification of the force and acceleration signals extracted during multiple machine processes: part 1 intelligent classification from an anomaly perspective
Artículo
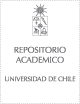
Open/ Download
Publication date
2017Metadata
Show full item record
Cómo citar
Griffin, James M.
Cómo citar
Multiple classification of the force and acceleration signals extracted during multiple machine processes: part 1 intelligent classification from an anomaly perspective
Author
Abstract
This paper is the first in a two-part work, where the investigation into the characteristics of multiple machine processes is made in order to accurately control them via the frequently used machine centre platform. The two machining processes under investigation are grinding and hole making: for grinding anomalies, grinding burn and chatter and for hole making, drilling, increased tool wear and onset of drill tool malfunction, which is also significant to severe scoring and material dragging. Most researchers usually report on one machining process as opposed to multiple which is less consistent with automated flexible systems where more than one machining process must be catered for. For efficient monitoring of automated multiple manufacturing processes, any unwanted anomalies should be identified and dealt with in a prompt and seamless manner. This first part provides two experimental set-ups (same set-up with tool interchange) to obtain signal signatures for both grinding and drilling phenomena (using the same material). Here, an approach based on neural networks and CARTs is used to reliably detect anomalies for both processes using a single acquisition path, opening the door for control implementation.
Indexation
Artículo de publicación ISI
Quote Item
Int J Adv Manuf Technol (2017) 93:811–823
Collections
The following license files are associated with this item: