Load estimation for microgrid planning based on a self-organizing map methodology
Artículo
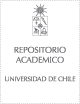
Open/Download
Publication date
2017Metadata
Show full item record
Cómo citar
Llanos, Jacqueline
Cómo citar
Load estimation for microgrid planning based on a self-organizing map methodology
Author
Abstract
tThis study presents a novel load estimation method for isolated communities that do not receive energyor only receive it for a limited time each day. These profiles have been used to determine the installedcapacity of generating units for microgrid electrification projects. The social characteristics and lifestylesof isolated communities differ from those in urban areas; therefore, the load profiles of microgrids aresensitive to minor variations in generation and/or consumption. The proposed methodology for obtainingthe residential profiles is based on clustering algorithms such as k-means, a self-organizing map (SOM) orothers. In this work, SOM clustering is considered because it allows a better interpretation of results thatcan be contrasted with social aspects. The proposed methodology includes the following components.First, the inputs are processed based on surveys of residents that live in each socio-economic level ofhousing and the community. Second, family types are clustered using an SOM, from which relevantinformation is derived that distinguishes one family from another. Third, the load profiles of each clusterare selected from a database. Additionally, social aspects and relevant energy supply information fromcommunities with similar characteristics are used to generate the required database. The SOM for theclustering of families of the community with available energy measurements is used as an initial guessfor the clustering of the families in the community with unknown energy measurements.The methodology is applied and tested in the community of El Romeral, Chile, where a microgrid willbe installed. The SOM technique compares favorably with a benchmark method that uses the averageload profile of a community; furthermore, the SOM clustering algorithm for the methodology is favorablycompared with the k-means algorithm because the results obtained by SOM are consistent with the socialaspects.
Indexation
Artículo de publicación SCOPUS
Identifier
URI: https://repositorio.uchile.cl/handle/2250/168793
DOI: 10.1016/j.asoc.2016.12.054
ISSN: 15684946
Quote Item
Applied Soft Computing 53 (2017) 323–335
Collections