Efficient Temporal Kernels Between Feature Sets for Time Series Classification
Artículo
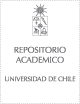
Open/ Download
Publication date
2017Metadata
Show full item record
Cómo citar
Tavenard, Romain
Cómo citar
Efficient Temporal Kernels Between Feature Sets for Time Series Classification
Author
Abstract
In the time-series classification context, the majority of themost accurate core methods are based on the Bag-of-Words framework,in which sets of local features are first extracted from time series. Adictionary of words is then learned and each time series is finally repre-sented by a histogram of word occurrences. This representation inducesa loss of information due to the quantization of features into words asall the time series are represented using the same fixed dictionary. Inorder to overcome this issue, we introduce in this paper a kernel operat-ing directly on sets of features. Then, we extend it to a time-compliantkernel that allows one to take into account the temporal information. Weapply this kernel in the time series classification context. Proposed kernelhas a quadratic complexity with the size of input feature sets, which isproblematic when dealing with long time series. However, we show thatkernel approximation techniques can be used to define a good trade-offbetween accuracy and complexity. We experimentally demonstrate thatthe proposed kernel can significantly improve the performance of timeseries classification algorithms based on Bag-of-Words.
Indexation
Artículo de publicación SCOPUS
Identifier
URI: https://repositorio.uchile.cl/handle/2250/169115
DOI: 10.1007/978-3-319-71246-8_32
ISSN: 16113349
03029743
Quote Item
Lecture Notes in Computer Science
LNCS, volume 10535, 2017
Collections