mrMoulder: A recommendation-based adaptive parameter tuning approach for big data processing platform
Artículo
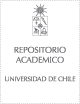
Open/ Download
Publication date
2019Metadata
Show full item record
Cómo citar
Cai, Lin
Cómo citar
mrMoulder: A recommendation-based adaptive parameter tuning approach for big data processing platform
Author
Abstract
© 2018 Elsevier B.V. Nowadays the world has entered the big data era. Big data processing platforms, such as Hadoop and Spark, are increasingly adopted by many applications, in which there are numerous parameters that can be tuned to improve processing performance for big data platform operators. However, due to the large number of these parameters and the complex relationship among them, it is very time-consuming to manually tune parameters. Therefore, it is a challenge to automatically configure parameters as quickly as possible to optimize the performance of the current job. Existing auto-tuning methods often take a certain time before job runs to get the optimal configuration, which would increase the job's total processing time and reduce the overall efficiency of cluster. In this paper, we propose an adaptive tuning framework, mrMoulder, to recommend a near-optimal configuration for the new job in a short time. mrMoulder sets a self-extending configuration repository and a collab
Indexation
Artículo de publicación SCOPUS
Identifier
URI: https://repositorio.uchile.cl/handle/2250/171366
DOI: 10.1016/j.future.2018.05.080
ISSN: 0167739X
Quote Item
Future Generation Computer Systems, Volumen 93,
Collections