System-level prognostics and health management: A graph convolutional network-based framework
Artículo
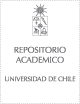
Open/ Download
Access note
Acceso a solo metadatos
Publication date
2020Metadata
Show full item record
Cómo citar
RuÍz-Tagle Palazuelos, Andrés
Cómo citar
System-level prognostics and health management: A graph convolutional network-based framework
Abstract
Sensing technologies have been used to gather massive amounts of data to improve system reliability analysis with the use of deep learning. Their use has been mainly focused on specific components or for the whole system, resulting in a drawback when dealing with complex systems as the interactions among components are not explicitly taken into account. Here, we propose a system-level prognostics and health management framework based on geometrical deep learning where a system, its components with their interactions, and sensor data are represented as a graph. This enables reliability analysis at different hierarchical levels by means of (1) a system-level module for system health diagnosis and prognosis based on embeddings of the system's learned features from a graph convolutional network; (2) a component-level module based on a deep graph convolutional network for health state diagnosis for the system's components; (3) a component interactions module based on a graph convolutional network autoencoder that allows for the identification of interactions among components when the system is in a degraded state. The framework is exemplified via a case study involving a chlorine dioxide generation system, in which it is shown that integrating both components' interactions and sensor data in the form of a graph improves health state diagnosis capabilities.
Patrocinador
Comisión Nacional de Investigación Científica y Tecnológica (CONICYT)
CONICYT FONDECYT
1190720
Indexation
Artículo de publicación ISI
Quote Item
Proceedings of the Institution of Mechanical Engineers, Part O: Journal of Risk and Reliability: Jul 2020
Collections