Pattern spotting in historical documents using convolutional models
Tesis
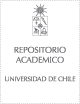
Publication date
2020Metadata
Show full item record
Cómo citar
Saavedra Rondo, José
Cómo citar
Pattern spotting in historical documents using convolutional models
Author
Professor Advisor
Abstract
Pattern spotting consists of locating different instances of a given object (i.e. an image query) in a collection of document images. Contrary to object detection, no prior information is given about the patterns that can be searched, hence no training can be done for localization. The queried patterns may vary in shape, size, color, context and even style, which makes pattern spotting a difficult task. To tackle this problem, we propose a convolutional neural network approach based on Feature Pyramid Networks (FPN) as the feature extractor of our system. Using FPN allows us to extract descriptors of local regions of the documents to be indexed and queries, at multiple scales with a single forward pass. Our main hypothesis is that deep features are more discriminatory than classical descriptors for pattern localization. Experiments conducted on DocExplore (a historical document dataset for pattern spotting evaluation) show
that the proposed system improves mAP by 73% (from 0.157 to 0.272) in pattern localization compared with state-of-the-art results, even when the feature extractor is not trained with domain-specific data. Memory requirement and computation time are also decreased since the descriptor dimension used for distance computation is reduced by a factor of 16. We conclude that CNN-based local descriptors are better than VLAD (classical) descriptors at locating patterns and we use them to propose a system for pattern localization. Limitation of our approach is that it struggles with non-square patterns. We also propose a solution to address this issue extracting multiple descriptors per query. Although it improves results in retrieving documents, it loses precision in locating patterns. Aggregation on those descriptors is proposed as interesting future
work in order to improve the system.
General note
Tesis para optar al grado de Magíster en Gestión de Operaciones Memoria para optar al título de Ingeniero Civil Industrial
Identifier
URI: https://repositorio.uchile.cl/handle/2250/177136
Collections
The following license files are associated with this item: