Condition-Based maintenance with reinforcement learning for dry gas pipeline subject to internal corrosión
Artículo
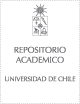
Open/ Download
Access note
Acceso Abierto
Publication date
2020Metadata
Show full item record
Cómo citar
Mahmoodzadeh, Zahra
Cómo citar
Condition-Based maintenance with reinforcement learning for dry gas pipeline subject to internal corrosión
Abstract
Gas pipeline systems are one of the largest energy infrastructures in the world and are known to be very efficient and reliable. However, this does not mean they are prone to no risk. Corrosion is a significant problem in gas pipelines that imposes large risks such as ruptures and leakage to the environment and the pipeline system. Therefore, various maintenance actions are performed routinely to ensure the integrity of the pipelines. The costs of the corrosion-related maintenance actions are a significant portion of the pipeline's operation and maintenance costs, and minimizing this large cost is a highly compelling subject that has been addressed by many studies. In this paper, we investigate the benefits of applying reinforcement learning (RL) techniques to the corrosion-related maintenance management of dry gas pipelines. We first address the rising need for a simulated testbed by proposing a test bench that models corrosion degradation while interacting with the maintenance decision-maker within the RL environment. Second, we propose a condition-based maintenance management approach that leverages a data-driven RL decision-making methodology. An RL maintenance scheduler is applied to the proposed test bench, and the results show that applying the proposed condition-based maintenance management technique can reduce up to 58% of the maintenance costs compared to a periodic maintenance policy while securing pipeline reliability.
Patrocinador
Petroleum Institute, Khalifa University of Science and Technology, Abu Dhabi, UAE
University of Maryland (Department of Mechanical Engineering)
Comision Nacional de Investigacion Cientifica y Tecnologica (CONICYT)
CONICYT FONDECYT
1190720
Indexation
Artículo de publicación ISI Artículo de publicación SCOPUS
Quote Item
Sensors (2020) 20:19
Collections
The following license files are associated with this item: