Automatic Crack Segmentation for UAV-Assisted Bridge Inspection
Artículo
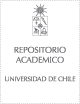
Open/ Download
Access note
Acceso Abierto
Publication date
2020Metadata
Show full item record
Cómo citar
Ayele, Yonas Zewdu
Cómo citar
Automatic Crack Segmentation for UAV-Assisted Bridge Inspection
Abstract
Bridges are a critical piece of infrastructure in the network of road and rail transport system. Many of the bridges in Norway (in Europe) are at the end of their lifespan, therefore regular inspection and maintenance are critical to ensure the safety of their operations. However, the traditional inspection procedures and resources required are so time consuming and costly that there exists a significant maintenance backlog. The central thrust of this paper is to demonstrate the significant benefits of adapting a Unmanned Aerial Vehicle (UAV)-assisted inspection to reduce the time and costs of bridge inspection and established the research needs associated with the processing of the (big) data produced by such autonomous technologies. In this regard, a methodology is proposed for analysing the bridge damage that comprises three key stages, (i) data collection and model training, where one performs experiments and trials to perfect drone flights for inspection using case study bridges to inform and provide necessary (big) data for the second key stage, (ii) 3D construction, where one built 3D models that offer a permanent record of element geometry for each bridge asset, which could be used for navigation and control purposes, (iii) damage identification and analysis, where deep learning-based data analytics and modelling are applied for processing and analysing UAV image data and to perform bridge damage performance assessment. The proposed methodology is exemplified via UAV-assisted inspection of Skodsberg bridge, a 140 m prestressed concrete bridge, in the Viken county in eastern Norway.
Patrocinador
Regionale Forskningsfond Oslofjordsfondet Norway
296349
Indexation
Artículo de publicación ISI Artículo de publicación SCOPUS
Quote Item
Energies 2020, 13, 6250
Collections
The following license files are associated with this item: