A continuous-time model of stochastic gradient descent: convergence rates and complexities under Lojasiewicz inequality
Tesis
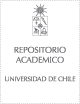
Publication date
2021Metadata
Show full item record
Cómo citar
Peypouquet Urbaneja, Juan
Cómo citar
A continuous-time model of stochastic gradient descent: convergence rates and complexities under Lojasiewicz inequality
Author
Professor Advisor
Abstract
In this thesis we study the convergence rates and complexities of a continuous model of the Stochastic Gradient Descent (SGD) under convexity, strong convexity and Łojasiewicz assumptions, the latter being a way to generalize the concept of strong convexity. In the first chapter, the concept of Łojasiewicz inequality is introduced and results of the Gradient Descent method in its discrete and continuous version are shown for this case, together with the convex and strongly convex cases. In the second chapter, the SGD method is introduced, results are shown under convex and strongly convex assumptions, and new results are obtained under the Łojasiewicz Inequality. Then it is shown how to construct a continuous-time model of SGD under a Gaussian variance assumption and, at last, the necessary concepts of Stochastic Analysis are introduced to understand this model. Finally, in the third chapter this model is analyzed and its upper bounds and complexities are obtained, where it is deduced that if the variance is sufficiently small, then the complexity of the model matches that of the Gradient Descent for the cases: convex, strongly convex and Łojasiewicz. En esta tesis se estudian las tasas de convergencia y complejidades de un modelo continuo del método del Gradiente Estocástico (SGD) bajo supuestos de convexidad, fuerte convexidad y Łojasiewicz, siendo este último una forma de generalizar el concepto de fuerte convexidad. En el primer capítulo se introduce el concepto de desigualdad de Łojasiewicz y se muestran resultados del método del Gradiente Descendente en su versión discreta y continua para este caso, junto con los casos convexo y fuertemente convexo. En el segundo capítulo se introduce el método de SGD, se muestran resultados bajo suposiciones de convexidad y fuerte convexidad, y se obtienen nuevos resultados bajo la desigualdad de Łojasiewicz. Luego se muestra como construir un modelo continuo de SGD bajo una suposición de varianza Gaussiana y por último se introducen los conceptos necesarios de Análisis Estocástico para comprender este modelo. Finalmente, en el tercer capítulo se analiza este modelo y se obtienen sus cotas superiores y complejidades, donde se deduce que si la varianza es suficientemente pequeña, entonces la complejidad del modelo coincide la del método del Gradiente Descendente para los casos: convexo, fuertemente convexo y Łojasiewicz.
General note
Tesis para optar al grado de Magíster en Ciencias de la Ingeniería, Mención Matemáticas Aplicadas Memoria para optar al título de Ingeniero Civil Matemático
Patrocinador
FONDECYT 1181179, ECOS 180039 Y CMM ANID PIA AFB170001
Identifier
URI: https://repositorio.uchile.cl/handle/2250/181234
Collections
The following license files are associated with this item: