An Apriori-Based Learning Scheme towards Intelligent Mining of Association Rules for Geological Big Data
Artículo
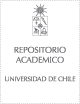
Open/ Download
Access note
Acceso Abierto
Publication date
2020Metadata
Show full item record
Cómo citar
Chen, Maojian
Cómo citar
An Apriori-Based Learning Scheme towards Intelligent Mining of Association Rules for Geological Big Data
Author
Abstract
The past decade has witnessed the rapid advancements of geological data analysis techniques, which facilitates the development of modern agricultural systems. However, there remains some technical challenges that should be addressed to fully exploit the potential of those geological big data, while gathering massive amounts of data in this application field. Generally, a good representation of correlation in the geological big data is critical to making full use of multi-source geological data, while discovering the relationship in data and mining mineral prediction information. Then, in this article, a scheme is proposed towards intelligent mining of association rules for geological big data. Firstly, we achieve word embedding via word2vec technique in geological data. Secondly, through the use of self-organizing map (SOM) and K-means algorithm, the word embedding data is clustered to serve the purpose of improving the performance of analysis and mining. On the basis of it, the unsupervised Apriori learning algorithm is developed to analyze and mine these association rules in data. Finally, some experiments are conducted to verify that our scheme can effectively mine the potential relationships and rules in the mineral deposit data.
Patrocinador
National Key Research and Development Program of China
2016YFC0600510
National Natural Science Foundation of China (NSFC)
U1836106
41872253
Beijing Natural Science Foundation
19L2029
Beijing Intelligent Logistics System Collaborative Innovation Center
BILSCIC-2019KF-08
Scientific and Technological Innovation Foundation of Shunde Graduate School, USTB
BK19BF006
Fundamental Research Funds for the University of Science and Technology Beijing
FRF-BD-19-012A
Indexation
Artículo de publicación ISI Artículo de publicación SCOPUS
Quote Item
IASC, 2020, vol.26, no.5
Collections
The following license files are associated with this item: