The effect of phased recurrent units in the classification of multiple catalogues of astronomical light curves
Artículo
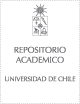
Access note
Acceso abierto
Publication date
2021Metadata
Show full item record
Cómo citar
Donoso Oliva, C.
Cómo citar
The effect of phased recurrent units in the classification of multiple catalogues of astronomical light curves
Abstract
In the new era of very large telescopes, where data is crucial to expand scientific knowledge, we have witnessed many deep
learning applications for the automatic classification of lightcurves. Recurrent neural networks (RNNs) are one of the models
used for these applications, and the LSTM unit stands out for being an excellent choice for the representation of long time series.
In general, RNNs assume observations at discrete times, which may not suit the irregular sampling of lightcurves. A traditional
technique to address irregular sequences consists of adding the sampling time to the network’s input, but this is not guaranteed
to capture sampling irregularities during training. Alternatively, the Phased LSTM unit has been created to address this problem
by updating its state using the sampling times explicitly. In this work, we study the effectiveness of the LSTM and Phased LSTM
based architectures for the classification of astronomical lightcurves. We use seven catalogs containing periodic and nonperiodic
astronomical objects. Our findings show that LSTM outperformed PLSTM on 6/7 datasets. However, the combination of both
units enhances the results in all datasets.
Patrocinador
ANID Millennium Science Initiative ICN12 009
Comision Nacional de Investigacion Cientifica y Tecnologica (CONICYT)
CONICYT FONDECYT 1171678
11191130
NLHPC ECM-02
Indexation
Artículo de publícación WoS
Quote Item
MNRAS 000, 1–17 (2015) Preprint 8 June 2021
Collections
The following license files are associated with this item: