Design of an image-based screening classifier for early detection of Parkinson's disease compatible patients using [18F]PR04. MZ Pet Tracer
Tesis
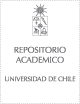
Access note
Acceso abierto
Publication date
2021Metadata
Show full item record
Cómo citar
Ríos Pérez, Sebastián
Cómo citar
Design of an image-based screening classifier for early detection of Parkinson's disease compatible patients using [18F]PR04. MZ Pet Tracer
Author
Professor Advisor
Abstract
Parkinsonism defines certain symptoms compatible with people who suffer from Parkinson's disease (PD), Essential Tremor, among other neurological disorders, present. Currently, PD is the second most important neurodegenerative pathology after Alzheimer. Today, the efforts of science are focused on knowing its causes and looking for biomarkers that facilitate an early diagnosis, as well as monitoring the evolutionary process of the disease due to the loss of dopamine in the substantia nigra pars compacta (SNpc).
In this context, clinicians can identify a patient compatible with PD by attending to motor and nonmotor manifestations. Motor symptoms remain as the core feature for diagnosis, defined as bradykinesia plus rest tremor or rigidity. Since a vast experience is required to give an accurate diagnosis, which still might not be error free, making it early may not always be possible, but certainly necessary. Once first symptoms have slightly appeared on stage one, the progression throughout all five stages of the disease is unstoppable. Although there's no cure, early treatments increase the chances of slowing dopamine deficiency, and therefore, delay the appearance of more severe symptoms.
The possibility of delaying the set of symptoms that characterizes last stages makes more urgent an early diagnosis, but doctors prefer to wait until the symptoms allow an accurate and differential diagnosis. Studies have proved that PD is directly related to the dopamine loss in the dopaminergic system, and therefore, detectable through molecular imaging techniques such as positron emission tomography (PET). The question is: what´s the probability of accuracy of pre-diagnose a patient compatible with PD by detecting the loss of dopamine neurons using machine learning (ML) and deep learning (DL) approaches on neuroimaging, that is, imaging processing?
With this finality, we used a sample of PET/CT images with [18F]PR04.MZ, a new PET imaging tracer, in order to diagnose the illness, and discussed the possibility of presenting a better precision using machine learning versus a movement-disorder specialist. The development of five models: Support Vector Machine (SVM), Random Forest, Logistic Regression, K-Nearest Neighbor (KNN) and Artificial Neural Network (ANN), allowed an extensive comparison between traditional classifies. Performances were greater than 96% for ML algorithms, and over 98% for the DL model, proving [18F]PR04.MZ tracer allows image-based algorithms have high precision for the identification of PD patterns.
xmlui.dri2xhtml.METS-1.0.item-notadetesis.item
Tesis para optar al grado de Magíster en Gestión de Operaciones
Identifier
URI: https://repositorio.uchile.cl/handle/2250/186902
Collections
The following license files are associated with this item: