Techniques of variational analysis: probability functions and estimators of non-convexity
Tesis
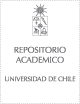
Access note
Acceso abierto
Publication date
2022Metadata
Show full item record
Cómo citar
Pérez Aros, Pedro
Cómo citar
Techniques of variational analysis: probability functions and estimators of non-convexity
Author
Professor Advisor
Abstract
This thesis aims to apply techniques of variational analysis to two different subjects: the first one being probability functions and the second one, a particular nonconvexity measure called effective standard deviation.
We approximate two different abstract formulations of probability functions. The first approximation is motivated by the fact that the constraints in optimization problems with uncertainty may result to be nonsmooth. We propose a regularization by applying the Moreau envelope to a scalar representation of a probability function consisting of a vector inequality, which covers most of the general classes of probabilistic constraints. We demostrate, under mild assumptions, the smoothness of such a regularization and that it satisfies a type of variational convergence to the original probability function. Consequently, when considering an appropriately structured problem involving probabilistic constraints, we can thus entail the convergence of the minimizers of the regularized approximate problems to the minimizers of the original problem. Finally, we illustrate our results with examples and applications in the field of (nonsmooth) joint, semidefinite and probust chance constrained optimization problems. The second formulation is a probability function generated by a set-valued mapping. Our main objective is to prove its local Lipschitz continuity. To do so, we propose an inner enlargement that, via the distance function, can be proven to be locally Lipschitz continuous. Subsequently, by approximation, we obtain our main result. As a consequence, we prove the local Lipschitz continuity of a Joint probability function given by a system of inequality constraints with a relaxed convexity assumption.
We recall that the projection operator onto closed convex subsets of Hilbert spaces is
single-valued. The converse is also true in finite-dimensional
Hilbert spaces, and also for weakly closed sets in any Hilbert space. This
is the famous Theorem of Klee. The problem of whether such a converse
holds in any Hilbert space for closed sets which are not weakly closed is
still unanswered. In this thesis, we apply variational characterizations of convexity results to the Asplund function to obtain a positive answer to this problem,
provided that the concept of projection is relaxed to the one of weak
projections. Finally, via the effective standard deviation measure, we estimate the Hausdorff distance between a set and its closed convex hull in terms of the size of the simultaneous projections on the set and its closed convex hull. Accordingly, we give a quantified version of Klee's theorem provided that the effective standard deviation of the set is finite.
This thesis ends with conclusions and future work.
xmlui.dri2xhtml.METS-1.0.item-notadetesis.item
Tesis para optar al grado de Doctora en Ciencias de la Ingeniería, Mención Modelación Matemática
Patrocinador
Agencia Nacional de Investigación y Desarrollo (ANID), Beca de Doctorado Nacional Chile/2017-21170428. Centro de Modelamiento Matemático (CMM) Proyecto ANID BASAL FB210005
Collections
The following license files are associated with this item: