Multi-object metrics for robotic map evaluation and data registration
Tesis
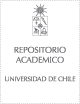
Access note
Acceso abierto
Publication date
2023Metadata
Show full item record
Cómo citar
Adams, Martin
Cómo citar
Multi-object metrics for robotic map evaluation and data registration
Author
Professor Advisor
Abstract
En mapeo robótico y en Simultaneous Localization And Mapping (SLAM), la capacidad de
evaluar la calidad de los mapas estimados es crucial. Aunque existen conceptos para cuantificar el error en la trayectoria estimada de un robot, o en un subconjunto de caracteristicas
del mapa estimado, rara vez se considera conjuntamente la diferencia entre todas las estimaciones y el ground truth. En la literatura de tracking, la métrica Optimal Sub-Pattern
Assignment (OSPA) proporciona una solución a este problema. A pesar de sus ventajas sobre
otras métricas, la métrica OSPA tiene algunas limitaciones en mapeo robótico. Por lo tanto,
la primera componente de esta tesis introduce la métrica Cardinalized Optimal Linear Assignment (COLA), para la evaluación de mapas robóticos. Además, la segunda componente
de esta tesis amplía el uso de las metricas multi-objeto al problema del registro de nubes de
puntos, el cual es una componente crucial en muchas aplicaciones, incluyendo la estimación
del movimiento de los sensores sensor y la reconstrucción 3D. Los resultados de esta tesis
demuestran que la métrica COLA es fiable para evaluar mapeo robótico y SLAM. Además,
la métrica COLA mejora el registro de nubes de puntos abordando conjuntamente los errores
espaciales y de cardinalidad. In robotic mapping and Simultaneous Localization And Mapping (SLAM) the ability to
assess the quality of estimated maps is crucial. While concepts exist for quantifying the error
in the estimated trajectory of a robot, or a subset of the estimated feature locations, the
difference between all current estimated and ground-truth features is rarely jointly considered.
The Optimal Sub-Pattern Assignment (OSPA) metric provided a solution to the problem of
assessing target tracking algorithms. Despite its advantages over other metrics when gauging
multi-target tracking errors, the OSPA metric has some limitations when applied to robotic
mapping errors. Therefore, the first component of this thesis introduces the Cardinalized
Optimal Linear Assignment (COLA) metric, as a complement to the OSPA metric, for feature
map evaluation Further, the point cloud registration problem is addressed as an application
of multi-object metric concepts. Therefore, the second component of this thesis extends the
application of multi-object metrics to the fundamental research problem of point cloud data
registration for sensor motion estimation and 3D reconstruction. The results of this thesis
demonstrate that the COLA metric is reliable for evaluating robotic mapping and SLAM.
Also, the COLA metric improves point cloud registration by jointly addressing spatial and
cardinality errors.
xmlui.dri2xhtml.METS-1.0.item-notadetesis.item
Tesis para optar al grado de Doctor en Ingeniería Eléctrica
Patrocinador
Becas CONICYT - Doctorado Nacional,
2012, ANID/PIA AFB180004 y por CONICYT FONDECYT project 1231658.
Identifier
URI: https://repositorio.uchile.cl/handle/2250/198815
Collections
The following license files are associated with this item: