Estimación de la densidad mamaria en imágenes de mamografía digital y tomosíntesis mediante inteligencia artificial
Tesis
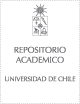
Open/ Download
Access note
Acceso abierto
Publication date
2023Metadata
Show full item record
Cómo citar
Castañeda, Víctor
Cómo citar
Estimación de la densidad mamaria en imágenes de mamografía digital y tomosíntesis mediante inteligencia artificial
Professor Advisor
Abstract
Antecedentes: La mamografía digital como método de screening ha tenido un gran impacto en la
detección precoz de cáncer de mama, sin embargo, han surgido otros métodos como la
tomosíntesis que han ido reemplazando esta técnica. La densidad mamaria es un factor de riesgo
para el cáncer de mama, es por esto su relevancia para cuantificarla con métodos automatizados.
En la actualidad, pocos estudios han estimado el porcentaje de densidad mamaria mediante
tomosíntesis y algoritmos de Deep learning. Objetivo: Desarrollar un algoritmo de inteligencia
artificial basado en Deep learning para la estimación del porcentaje de densidad mamaria en
imágenes de tomosíntesis y mamografía digital, en las proyecciones craneocaudal (CC) y
mediolateral oblicua (MLO). Materiales y método: Se escogió una muestra de 19 exámenes de
tomosíntesis mamaria y 81 de mamografía digital, ambos provenientes de bases de datos
públicas y anonimizadas. En ellos se realizó una segmentación manual con el software 3D Slicer
y posteriormente se desarrolló un algoritmo de Deep learning utilizando el entorno de trabajo
MONAI para la estimación del porcentaje de densidad mamaria. Resultados: El rendimiento del
algoritmo alcanzó para el conjunto de entrenamiento/validación un Dice promedio de 0.8041 y
0.8793 para tomosíntesis y mamografía, respectivamente, y para el conjunto de prueba un
0.8157 para tomosíntesis mamaria y 0.8452 para mamografía. Existió correlación entre los
etiquetados manual y automatizado en las proyecciones para ambos exámenes (p < 0.001) en el
conjunto de entrenamiento/validación. La mayor correlación ocurre en las proyecciones CC para
ambos exámenes. Al comparar el porcentaje de densidad mamaria en mamografía y tomosíntesis
se obtuvieron diferencias significativas entre los dos grupos, con mayor valor para mamografía.
Conclusiones: El algoritmo creado con Deep Learning para estimar la densidad mamaria en
mamografía y tomosíntesis tuvo un buen desempeño tanto en proyecciones CC como MLO. Se
requiere validar sus resultados con imágenes de resonancia magnética mamaria. Background: Digital mammography as a screening method has had a great impact on the
early detection of breast cancer, however, other methods such as tomosynthesis have been
replacing this technique. Breast density is a risk factor for breast cancer, which is why it is so
important to quantify it with automated methods. At present, few studies have estimated the
percentage of breast density using tomosynthesis and deep learning algorithms.Purpose: To
develop an artificial intelligence algorithm based on deep learning for the estimation of the
percentage of breast density in tomosynthesis and digital mammography images, in
craniocaudal (CC) and mediolateral oblique (MLO) projections.Materials and method: A
sample of 19 breast tomosynthesis examinations and 81 digital mammography examinations,
both from public and anonymized databases, were chosen. A manual segmentation was
performed with 3D Slicer software and then a deep learning algorithm was developed using
the MONAI framework to estimate the percentage of breast density. Results: The algorithm
performance reached for the training/validation set an average Dice of 0.8041 and 0.8793 for
tomosynthesis and mammography, respectively, and for the test set 0.8157 for mammary
tomosynthesis and 0.8452 for mammography. There was correlation between manual and
automated labeling in the projections for both examinations (p < 0.001) in the
training/validation set. The highest correlation occurs in the CC projections for both exams.
When comparing the percentage of breast density in mammography and tomosynthesis,
significant differences were obtained between the two groups, with a higher value for
mammography. Conclusions: The algorithm created with Deep Learning to estimate breast
density in mammography and tomosynthesis performed well in both CC and MLO projections.
It is required to validate its results with breast magnetic resonance images.
xmlui.dri2xhtml.METS-1.0.item-notadetesis.item
Tesis para optar al título profesional de Tecnóloga Médica con mención en Imagenología, Radioterapia y Física Médica
Patrocinador
NLHPC (CCSS210001)
Collections
The following license files are associated with this item: