Multilingual hate speech detection
Tesis
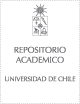
Open/ Download
Access note
Acceso abierto
Publication date
2024
Author
Professor Advisor
Abstract
El crecimiento de las plataformas web sociales en los últimos años ha llevado a un aumento del discurso de odio en línea. Este tema es considerado crítico en la comunidad web, ya que puede estar relacionado con acciones peligrosas que afectan a individuos y grupos en el mundo real. Por lo tanto, los algoritmos de moderación automática son una herramienta necesaria.
A pesar del creciente interés en esta área de investigación, la mayoría de la literatura relacionada describe enfoques para el idioma inglés. Como consecuencia, la mayoría de los conjuntos de datos anotados y recursos disponibles están en este idioma. La construcción de estos recursos es costosa en tiempo y esfuerzo. En lugar de crear recursos para todos los demás idiomas uno por uno, sería útil tener estrategias que pudieran aplicarse a diferentes idiomas y dominios. Una limitante para una estrategia de aprendizaje por transferencia es la escasa capacidad de generalización de los modelos existentes. Los modelos de detección de discursos de odio muestran un alto rendimiento en evaluaciones dentro de un mismo conjunto de datos, pero no generalizan bien a otros, lo que los hace inútiles en escenarios reales.
El objetivo de nuestra investigación es entender y contribuir a la tarea de detección de discursos de odio. Proponemos abordar la tarea mediante estrategias de generalización para usar los recursos existentes en los idiomas poco tratados.
En esta tesis, describimos nuestras contribuciones al área de detección de discursos de odio multilingües. Mostramos un panorama realista del estado del arte en la tarea en escenarios monolingües en inglés, destacando los desafíos de abordar la tarea en diferentes idiomas. Proponemos diferentes conjuntos de características multilingües o independientes del lenguaje para la detección de discursos de odio. Diseñamos un modelo para aprovechar varias fuentes de datos al mismo tiempo y creamos el primer conjunto de datos chileno etiquetado para la detección de lenguaje ofensivo y discurso de odio. The growth in social Web platforms in the past years has brought an increase in displays of
online hate speech. This subject is considered a critical matter in the Web community since
it can be related to potentially dangerous actions that affect individuals and groups in the
physical world. This kind of speech is present in online environments, and in addition to
the evident discomfort that this kind of comment provokes in public virtual spaces, it brings
with it the bigger risk of encouraging real hate crimes. Therefore, automatic moderation
algorithms are a necessary tool.
Despite the growing interest in this research area, most related literature describes approaches for the English language. As a consequence, most of the available annotated datasets
and resources are in this language. The construction of such resources is costly in time and
effort. Instead of creating resources for all other languages one by one, it would be useful
to have strategies that could be applied across different languages and domains. A limiting
reason for a transferring learning strategy is the poor generalization ability of the existing
models. Hate speech detection models show high performance in intra-dataset evaluations
but they do not generalize well to others which makes them useless in real scenarios.
The goal of our research is to understand and contribute to the task of hate speech
detection. We propose to approach the task through generalization strategies to use the
existing monolingual resources in the under-represented languages.
In this thesis, we describe our contributions to the multilingual hate speech detection
area. We show a realistic picture of the state of the art on the task in monolingual English
scenarios while highlighting the challenges of tackling the task in different languages. We
propose two sets of features for multilingual hate speech detection. The first set of features
is extracted from network meta-information, and the second is a set of word embeddings
leveraged specifically for the task of hate speech detection. We designed a model for taking
advantage of several data sources at the same time. In addition, we constructed some useful
resources for future research on this topic. One of them is the first Chilean dataset labeled
for offensive language and hate speech detection and a data repository where we organized
multilingual resources for the task.
xmlui.dri2xhtml.METS-1.0.item-notadetesis.item
Tesis para optar al grado de Doctora en Computación
Identifier
URI: https://repositorio.uchile.cl/handle/2250/204835
Collections
The following license files are associated with this item: