Can machines analyze discourse beyond sentiment analysis? : automating linguistic analyses of attitude and negative judgment
Tesis
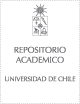
Access note
Acceso abierto
Publication date
2024Metadata
Show full item record
Cómo citar
Bravo Márquez, Felipe
Cómo citar
Can machines analyze discourse beyond sentiment analysis? : automating linguistic analyses of attitude and negative judgment
Author
Professor Advisor
Abstract
Esta investigación aborda la automatización del análisis lingüístico de Actitud según la Teoría de la Valoración de la Lingüística Sistémico Funcional (SFL). Los objetivos comprenden el etiquetado de un corpus en español de Chile con el análisis de Actitud, con énfasis en los Juicios negativos, y la automatización computacional de estos análisis. Este trabajo apuesta a reducir las brechas entre los métodos computacionales y los análisis del discurso realizados por humanos.
La metodología incluye la selección de corpus, anotación y desarrollo de modelos. Construimos un corpus etiquetado de textos en español chileno, centrado en juicios negativos, anotado por tres lingüistas expertos para asegurar la concordancia entre anotadores. La anotación se dividió en dos campañas: identificación de grupos nominales para delimitar mejor el problema y etiquetado de Tipos de Actitud, Tipos de Juicio y Subtipos de Juicio.
La automatización del análisis se formuló como tres tareas de Sequence Labeling: a nivel de Tipos de Actitud, a nivel de Tipos de Juicio y a nivel de Subtipo de Juicio. Utilizamos tres arquitecturas de Machine Learning: redes Long Short-Term Memory (LSTM), modelos basados en Transformer y Few-shot Learning con Modelos de Lenguaje Generativos (LLMs). Evaluamos los modelos con métricas de precisión, recall y puntaje F1.
Los resultados muestran que, aunque los modelos de aprendizaje automático usados pueden generalizar la detección de Actitud y Juicios negativos, su rendimiento no alcanzó la precisión humana. Los modelos basados en Transformer destacaron en clasificaciones de categorías más amplias, logrando un puntaje F1 de $0.510$ para la clasificación de Actitud. Los modelos LSTM tuvieron un mejor desempeño en categorías más detalladas, con puntajes F1 de $0.579$ y $0.392$ para Tipos y Subtipos de Juicio, respectivamente. En cambio, el few shot learning con LLMs mostró potencial pero no tuvo un rendimiento tan bueno como los modelos de Deep Learning.
Concluimos que la automatización del análisis de Actitud y Juicios negativos es factible pero desafiante, debido a la subjetividad y dependencia contextual del lenguaje humano. Esta investigación aporta con el primer corpus público de textos en español chileno anotados para Actitud y con metodologías para la anotación de datos y entrenamiento de modelos. Los hallazgos subrayan la necesidad de más investigación para cerrar la brecha de rendimiento entre humanos y máquinas. This research addresses the automation of linguistic analysis of Attitude, as defined by Systemic Functional Linguistics (SFL) Appraisal Theory. The objectives encompass the annotation of a Chilean Spanish corpus with Attitude analysis, with an emphasis on negative
Judgments, and the computational automation of these analyses. This work aims to bridge
the gap between computational methods and discourse analysis performed by humans.
The methodology includes corpus selection, annotation, and model development. We constructed a labeled corpus of Chilean Spanish texts, focused on negative judgments, annotated
by three expert linguists to ensure inter-annotator agreement. The annotation was divided
into two campaigns: the identification of nominal groups to better delimit the problem, and
the labeling of Attitude Types, Judgment Types, and Judgment Subtypes.
The automation of the analysis was formulated as three Sequence Labeling tasks: at the
Attitude Type level, at the Judgment Type level, and at the Judgment Subtype level. We
utilized three Machine Learning architectures: Long Short-Term Memory (LSTM) networks,
Transformer-based models, and few-shot learning with Generative Language Models (LLMs).
We evaluated the models using precision, recall, and F1-score metrics.
The results show that, although Machine Learning models used can generalize the detection of Attitude and negative Judgments, their performance does not reach human precision.
Transformer-based models excelled in broader category classifications, achieving an F1-score
of 0.510 for Attitude classification. LSTM models performed better in more detailed categories, with F1-scores of 0.579 and 0.392 for Judgment Types and Subtypes, respectively. In
contrast, few-shot learning with LLMs showed potential but did not perform as well as Deep
Learning models.
We conclude that the automation of Attitude and negative Judgment analysis is feasible
but challenging, due to the subjectivity and contextual dependence of human language. This
research contributes the first public corpus of Chilean Spanish texts annotated for Attitude,
as well as methodologies for data annotation and model training. The findings underscore
the need for further research to bridge the performance gap between humans and machines
xmlui.dri2xhtml.METS-1.0.item-notadetesis.item
Tesis para optar al grado de Magíster en Ciencias de la Computación
Patrocinador
Este trabajo ha sido parcialmente financiado por The Millennium Institute Foundational
Research on Data
Identifier
URI: https://repositorio.uchile.cl/handle/2250/205045
Collections
The following license files are associated with this item: