Predicting Vascular Plant Richness in a Heterogeneous Wetland Using Spectral and Textural Features and a Random Forest Algorithm
Artículo
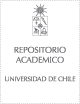
Open/ Download
Publication date
2016Metadata
Show full item record
Cómo citar
Cabezas, Julián
Cómo citar
Predicting Vascular Plant Richness in a Heterogeneous Wetland Using Spectral and Textural Features and a Random Forest Algorithm
Abstract
A method to predict vascular plant richness using spectral and textural variables in a heterogeneous wetland is presented. Plant richness was measured at 44 sampling plots in a 16-ha anthropogenic peatland. Several spectral indices, first-order statistics (median and standard deviation), and second-order statistics [metrics of a gray-level co-occurrence matrix (GLCM)] were extracted from a Landsat 8 Operational Land Imager image and a Pleiades 1B image. We selected the most important variables for predicting richness using recursive feature elimination and then built a model using random forest regression. The final model was based on only two textural variables obtained from the GLCM and derived from the Landsat 8 image. An accurate predictive capability was reported (R-2 = 0.6; RMSE = 1.99 species), highlighting the possibility of obtaining parsimonious models using textural variables. In addition, the results showed that the mid-resolution Landsat 8 image provided better predictors of richness than the high-resolution Pleiades image. This is the first study to generate a model for plant richness in a wetland ecosystem.
Patrocinador
CONICYT through the FONDECYT
1130935
Indexation
Artículo de publicación ISI
Quote Item
IEEE Geoscience and Remote Sensing Letters, Vol. 13, No. 5, May 2016
Collections
The following license files are associated with this item: