Deep learning enabled fault diagnosis using time-frequency image analysis of rolling element bearings
Artículo
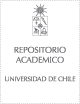
Open/ Download
Date
2017Metadata
Show full item record
Cómo citar
Verstraete, David
Cómo citar
Deep learning enabled fault diagnosis using time-frequency image analysis of rolling element bearings
Author
Abstract
Traditional feature extraction and selection is a labor-intensive process requiring expert knowledge of the relevant features pertinent to the system. This knowledge is sometimes a luxury and could introduce added uncertainty and bias to the results. To address this problem a deep learning enabled featureless methodology is proposed to automatically learn the features of the data. Time-frequency representations of the raw data are used to generate image representations of the raw signal, which are then fed into a deep convolutional neural network (CNN) architecture for classification and fault diagnosis. This methodology was applied to two public data sets of rolling element bearing vibration signals. Three time-frequency analysis methods (short-time Fourier transform, wavelet transform, and Hilbert-Huang transform) were explored for their representation effectiveness. The proposed CNN architecture achieves better results with less learnable parameters than similar architectures used for fault detection, including cases with experimental noise.
Patrocinador
Chilean National Fund for Scientific and Technological Development (Fondecyt) 1160494
Indexation
Artículo de publicación ISI
Quote Item
Shock and Vibration Vol 2017, Article ID 5067651
Collections
The following license files are associated with this item: