Redefining support vector machines with the ordered weighted average
Artículo
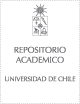
Open/ Download
Publication date
2018Metadata
Show full item record
Cómo citar
Maldonado, Sebastián
Cómo citar
Redefining support vector machines with the ordered weighted average
Abstract
In this work, the classical soft-margin Support Vector Machine (SVM) formulation is redefined with the inclusion of an Ordered Weighted Averaging (OWA) operator. In particular, the hinge loss function is rewritten as a weighted sum of the slack variables to guarantee adequate model fit. The proposed twostep approach trains a soft-margin SVM first to obtain the slack variables, which are then used to induce the order for the OWA operator in a second SVM training. Originally developed as a linear method, our proposal extends it to nonlinear classification thanks to the use of Kernel functions. Experimental results show that the proposed method achieved the best overall performance compared with standard SVM and other well-known data mining methods in terms of predictive performance.
Patrocinador
CONICYT
FONDECYT
1160286
1160738
Complex Engineering Systems Institute (CONICYT, PIA)
FB0816
Indexation
Artículo de publicación ISI
Quote Item
Knowledge-Based Systems, 148 (2018): 41–46
Collections
The following license files are associated with this item: