Embedded heterogeneous feature selection for conjoint analysis: A SVM approach using L1 penalty
Artículo
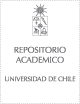
Open/ Download
Publication date
2017Metadata
Show full item record
Cómo citar
Maldonado, Sebastián
Cómo citar
Embedded heterogeneous feature selection for conjoint analysis: A SVM approach using L1 penalty
Abstract
This paper presents a novel embedded feature selection approach for Support Vector Machines (SVM) in a choice-based conjoint context. We extend the L1-SVM formulation and adapt the RFE-SVM algorithm to conjoint analysis to encourage sparsity in consumer preferences. This sparsity can be attributed to consumers being selective about the attributes they consider when evaluating alternatives in choice tasks. Given limited individual data in choice-based conjoint, we control for heterogeneity by pooling information across consumers and shrinking the individual weights of the relevant attributes towards a population mean. We tested our approach through an extensive simulation study that shows that the proposed approach can capture the sparseness implied by irrelevant attributes. We also illustrate the characteristics and use of our approach on two real-world choice-based conjoint data sets. The results show that the proposed method has better predictive accuracy than competitive approaches, and that it provides additional information at an individual level. Implications for product design decisions are discussed.
Indexation
Artículo de publicación SCOPUS
Identifier
URI: https://repositorio.uchile.cl/handle/2250/168823
DOI: 10.1007/s10489-016-0852-5
ISSN: 15737497
0924669X
Quote Item
Applied Intelligence, June 2017, Volume 46, Issue 4, pp 775–787
Collections