Emergency department readmission risk prediction: A case study in Chile
Artículo
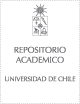
Publication date
2017Metadata
Show full item record
Cómo citar
Artetxe, Arkaitz
Cómo citar
Emergency department readmission risk prediction: A case study in Chile
Abstract
Short time readmission prediction in Emergency Depart-ments (ED) is a valuable tool to improve both the ED managementand the healthcare quality. It helps identifying patients requiring fur-ther post-discharge attention as well as reducing healthcare costs. As inmany other medical domains, patient readmission data is heavily imbal-anced, i.e. the minority class is very infrequent, which is a challenge forthe construction of accurate predictors using machine learning tools. Wehave carried computational experiments on a dataset composed of EDadmission records spanning more than 100000 patients in 3 years, witha highly imbalanced distribution. We employed various approaches fordealing with this highly imbalanced dataset in combination with differ-ent classification algorithms and compared their predictive power for theestimation of the ED readmission probability within 72 h after discharge.Results show that random undersampling and Bagging (RUSBagging) incombination with Random Forest achieves the best results in terms ofArea Under ROC Curve (AUC).
Indexation
Artículo de publicación SCOPUS
Identifier
URI: https://repositorio.uchile.cl/handle/2250/169031
DOI: 10.1007/978-3-319-59773-7_2
ISSN: 16113349
03029743
Quote Item
Lecture Notes in Computer Science , Volumen 10338 LNCS, 2017
Collections