Spectral regularization in generalized matrix learning vector quantization
Artículo
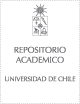
Publication date
2017Metadata
Show full item record
Cómo citar
Nova, David
Cómo citar
Spectral regularization in generalized matrix learning vector quantization
Author
Abstract
In this contribution we propose a new regularization method for the Generalized Matrix Learning Vector Quantization classifier. In particular we use a nuclear norm in order to prevent oversimplifying/over-fitting and oscillatory behaviour of the small eigenvalues of the positive semi-definite relevance matrix. The proposed method is compared with two other regularization methods in two artificial data sets and a real-life problem. The results show that the proposed regularization method enhances the generalization ability of GMLVQ. This is reflected in a lower classification error and a better interpretability of the relevance matrix.
Indexation
Artículo de publicación SCOPUS
Collections