Addressing Data Association in Maximum Likelihood SLAM with Random Finite Sets
Artículo
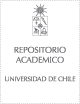
Open/ Download
Publication date
2018Metadata
Show full item record
Cómo citar
Opazo Inostroza, Felipe
Cómo citar
Addressing Data Association in Maximum Likelihood SLAM with Random Finite Sets
Author
Abstract
Recently, various algorithms which adopt Random Finite Sets (RFS) for the solution of the fundamental, autonomous robotic, feature based, Simultaneous Localization and Mapping (SLAM) problem, have been proposed. In contrast to their vector based counterparts, these techniques jointly estimate the vehicle and map state and map cardinality. Most of the proposed RFS solutions are based on a Rao-Blackwellized particle filter representing the vehicle state, accompanied by an RFS filter to represent the map. This article shows that an RFS maximum likelihood approach to SLAM is also possible. By maximizing the RFS based measurement likelihood this article demonstrates that Maximum Likelihood (ML) SLAM is possible without the need for external data association algorithms. It will be demonstrated that RFS based ML-SLAM converges to the same solution as its traditional vector-based counterpart. However, fundamentally RFS-ML-SLAM does not require the correct data association decisions necessary for the correct convergence of traditional random vector based approaches.
Indexation
Artículo de publicación SCOPUS
Quote Item
21st International Conference on Information Fusion (FUSION) (2018). Pages 2047-2053.
Collections