On variance reduction for stochastic smooth convex optimization with multiplicative noise
Artículo
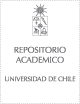
Open/ Download
Publication date
2019Metadata
Show full item record
Cómo citar
Jofré Cáceres, René
Cómo citar
On variance reduction for stochastic smooth convex optimization with multiplicative noise
Author
Abstract
© 2018, Springer-Verlag GmbH Germany, part of Springer Nature and Mathematical Optimization Society. We propose dynamic sampled stochastic approximation (SA) methods for stochastic optimization with a heavy-tailed distribution (with finite 2nd moment). The objective is the sum of a smooth convex function with a convex regularizer. Typically, it is assumed an oracle with an upper bound σ 2 on its variance (OUBV). Differently, we assume an oracle with multiplicative noise. This rarely addressed setup is more aggressive but realistic, where the variance may not be uniformly bounded. Our methods achieve optimal iteration complexity and (near) optimal oracle complexity. For the smooth convex class, we use an accelerated SA method a la FISTA which achieves, given tolerance ε> 0 , the optimal iteration complexity of O(ε-12) with a near-optimal oracle complexity of O(ε-2)[ln(ε-12)]2. This improves upon Ghadimi and Lan (Math Program 156:59–99, 2016) where it is assumed an OUBV. For the strongly
Indexation
Artículo de publicación SCOPUS
Identifier
URI: https://repositorio.uchile.cl/handle/2250/171301
DOI: 10.1007/s10107-018-1297-x
ISSN: 14364646
00255610
Quote Item
Mathematical Programming, Volumen 174, Issue 1-2, 2019, Pages 253-292
Collections