Deep-learning-based physical layer authentication for industrial wireless sensor networks
Artículo
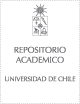
Open/ Download
Access note
Acceso Abierto
Publication date
2019Metadata
Show full item record
Cómo citar
Liao, Run Fa
Cómo citar
Deep-learning-based physical layer authentication for industrial wireless sensor networks
Author
Abstract
In this paper, a deep learning (DL)-based physical (PHY) layer authentication framework is proposed to enhance the security of industrial wireless sensor networks (IWSNs). Three algorithms, the deep neural network (DNN)-based sensor nodes’ authentication method, the convolutional neural network (CNN)-based sensor nodes’ authentication method, and the convolution preprocessing neural network (CPNN)-based sensor nodes’ authentication method, have been adopted to implement the PHY-layer authentication in IWSNs. Among them, the improved CPNN-based algorithm requires few computing resources and has extremely low latency, which enable a lightweight multi-node PHY-layer authentication. The adaptive moment estimation (Adam) accelerated gradient algorithm and minibatch skill are used to accelerate the training of the neural networks. Simulations are performed to evaluate the performance of each algorithm and a brief analysis of the application scenarios for each algorithm is discussed. Moreover, the experiments have been performed with universal software radio peripherals (USRPs) to evaluate the authentication performance of the proposed algorithms. Due to the trainings being performed on the edge sides, the proposed method can implement a lightweight authentication for the sensor nodes under the edge computing (EC) system in IWSNs.
Indexation
Artículo de publicación SCOPUS
Identifier
URI: https://repositorio.uchile.cl/handle/2250/172274
DOI: 10.3390/s19112440
ISSN: 14248220
Quote Item
Sensors (Switzerland), Volumen 19, Issue 11, 2019,
Collections