Gender Classification from NIR Images by Using Quadrature Encoding Filters of the Most Relevant Features
Artículo
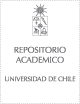
Open/ Download
Access note
Acceso Abierto
Date
2019Metadata
Show full item record
Cómo citar
Tapia, Juan E.
Cómo citar
Gender Classification from NIR Images by Using Quadrature Encoding Filters of the Most Relevant Features
Author
Abstract
In the past few years, accuracy in determining gender from iris images has increased significantly, approaching levels that make novel applications of this biometric technology feasible. In this paper, we report the gender classification rate by using a 2-D Quadrature Quaternionic filter, and a selection of the most relevant features from the normalized iris images. We encoded the phase information of the normalized images using 4 bits per pixel with a 2-D-Gabor filter and selected the best bits from the four resulting images (1 real and 3 imaginary) instead of the 1-D log-Gabor traditional encoding method. We used traditional hand-crafted and automatic methods to select and extract the most relevant features from the whole iris images, blocks from images, and pixel features and compared how effective these methods were in separating features from female and male iris images. Selecting iris blocks and features reduce the computational time and, at a basic science level, is of great value in understanding what information features, as well as pixels from the iris, can be extracted to classify gender. The Quaternionic-Code with the complementary feature selection method achieved the best results on the GFI-UND database with 93.45% for the left iris and 95.45% for the right iris, both with 2400 selected features. We compared our results and found them to be advantageous to the best results previously published, and also to those obtained using convolutional neural network feature extraction.
Indexation
Artículo de publicación SCOPUS
Identifier
URI: https://repositorio.uchile.cl/handle/2250/172382
DOI: 10.1109/ACCESS.2019.2902470
ISSN: 21693536
Quote Item
IEEE Access, Volumen 7,
Collections