Profit-based churn prediction based on Minimax Probability Machines
Artículo
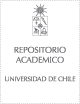
Open/ Download
Access note
Acceso Abierto
Publication date
2020Metadata
Show full item record
Cómo citar
Maldonado, Sebastián
Cómo citar
Profit-based churn prediction based on Minimax Probability Machines
Author
Abstract
In this paper, we propose three novel profit-driven strategies for churn prediction. Our proposals extend the ideas of the Minimax Probability Machine, a robust optimization approach for binary classification that maximizes sensitivity and specificity using a probabilistic setting. We adapt this method and other variants to maximize the profit of a retention campaign in the objective function, unlike most profit-based strategies that use profit metrics to choose between classifiers, and/or to define the optimal classification threshold given a probabilistic output. A first approach is developed as a learning machine that does not include a regularization term, and subsequently extended by including the LASSO and Tikhonov regularizers. Experiments on well-known churn prediction datasets show that our proposal leads to the largest profit in comparison with other binary classification techniques.
Patrocinador
Comisión Nacional de Investigación Científica y Tecnológica (CONICYT)
CONICYT PIA/BASAL
AFB180003
Comisión Nacional de Investigación Científica y Tecnológica (CONICYT)
CONICYT FONDECYT
1160738
1160894
Indexation
Artículo de publicación ISI Artículo de publicación SCOPUS
Quote Item
European Journal of Operational Research 284 (2020) 273–284
Collections
The following license files are associated with this item: