Feature detection with a constant FAR in sparse 3-D point cloud data
Artículo
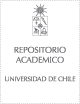
Open/ Download
Access note
Acceso Abierto
Publication date
2020Metadata
Show full item record
Cómo citar
Lühr, Daniel
Cómo citar
Feature detection with a constant FAR in sparse 3-D point cloud data
Abstract
The detection of markers or reflectors within point cloud data (PCD) is often used for 3-D scan registration, mapping, and 3-D environmental modeling. However, the reliable detection of such artifacts is diminished when PCD is sparse and corrupted by detection and spatial errors, for example, when the sensing environment is contaminated by high dust levels, such as in mines. In the radar literature, constant false alarm rate (CFAR) processors provide solutions for extracting features within noisy data; however, their direct application to sparse, 3-D PCD is limited due to the difficulty in defining a suitable noise window. Therefore, in this article, CFAR detectors are derived, which are capable of processing a 2-D projected version of the 3-D PCD or which can directly process the 3-D PCD itself. Comparisons of their robustness, with respect to data sparsity, are made with various state-of-the-art feature detection methods, such as the Canny edge detector and random sampling consensus (RANSAC) shape detection methods.
Patrocinador
Comision Nacional de Investigacion Cientifica y Tecnologica (CONICYT)
AFB180004
Comision Nacional de Investigacion Cientifica y Tecnologica (CONICYT)
CONICYT FONDECYT
1190979
CONICYT-Deutscher Akademischer Austauchdienst (DAAD), ChileGermany Collaborative Grant, through Automated 3D Scan Acquisition for fast Digitization of Mines
DAAD PCCI12009/56088171
Indexation
Artículo de publicación ISI Artículo de publicación SCOPUS
Quote Item
IEEE Transactions on Geoscience and Remote Sensing, 58 (3): 1877-1891, 2020
Collections
The following license files are associated with this item: