Operation scheduling in a solar thermal system: A reinforcement learning-based framework
Artículo
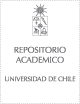
Access note
Acceso Abierto
Publication date
2020Metadata
Show full item record
Cómo citar
Correa Jullian, Camila
Cómo citar
Operation scheduling in a solar thermal system: A reinforcement learning-based framework
Abstract
Reinforcement learning (RL) provides an alternative method for designing condition-based decision making in engineering systems. In this study, a simple and flexible RL tabular Q-learning framework is employed to identify the optimal operation schedules for a solar hot water system according to action-reward feedback. The system is simulated in TRNSYS software. Three energy sources must supply a building's hot-water demand: low-cost heat from solar thermal collectors and a heat-recovery chiller, coupled to a conventional heat pump. Key performance indicators are used as rewards for balancing the system's performance with regard to energy efficiency, heat-load delivery, and operational costs. A sensitivity analysis is performed for different reward functions and meteorological conditions. Optimal schedules are obtained for selected scenarios in January, April, July, and October, according to the dynamic conditions of the system. The results indicate that when solar radiation is widely available (October through April), the nominal operation schedule frequently yields the highest performance. However, the obtained schedule differs when the solar radiation is reduced, for instance, in July. On average, with prioritization of the efficient use of both low-cost energy sources, the performance in July can be on average 21% higher than under nominal schedule-based operation.
Patrocinador
ANID/FONDAP "Solar Energy Research Center" SERC-Chile
15110019
Comision Nacional de Investigacion Cientifica y Tecnologica (CONICYT)
CONICYT FONDECYT
1190720
Indexation
Artículo de publicación ISI Artículo de publicación SCOPUS
Identifier
URI: https://repositorio.uchile.cl/handle/2250/175738
DOI: 10.1016/j.apenergy.2020.114943
Quote Item
Applied Energy 268 (2020) 114943
Collections
The following license files are associated with this item: