Deep learning health state prognostics of physical assets in the Oil and Gas industry
Artículo
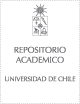
Open/ Download
Access note
Acceso a solo metadatos
Date
2020Metadata
Show full item record
Cómo citar
Figueroa Barraza, Joaquín Eduardo
Cómo citar
Deep learning health state prognostics of physical assets in the Oil and Gas industry
Author
Abstract
Due to its capital-intensive nature, the Oil and Gas industry requires high operational standards to meet safety and environmental requirements, while maintaining economical returns. In this context, maintenance policies play a crucial role in the avoidance of unplanned downtimes and enhancement of productivity. In particular, Condition-Based Maintenance is an approach in which maintenance actions are performed depending on the assets' health state that is evaluated through different kinds of sensors. In this paper, Deep Learning methods are explored and different models are proposed for health state prognostics of physical assets in two real-life cases from the Oil and Gas industry: a Natural Gas treatment plant in an offshore production platform where elevated levels of CO2 must be predicted, and a sea water injection pump for oil extraction stimulation, in which several degradation levels must be predicted. A general methodology for preprocessing the available multi-sensor data and developing proper models is proposed and apply in both case studies. In the first one, a LSTM autoencoder is developed, achieving precision values over 83.5% when predicting anomalous states up to 8 h ahead. In the second case study, a CNN-LSTM model is proposed for the pump's health state prognostics 48 h ahead, achieving precision values above 99% for all possible pump health states.
Patrocinador
Conselho Nacional de Desenvolvimento Cientifico e Tecnologico
(CNPQ)
308712/2019-6
Indexation
Artículo de publicación ISI
Quote Item
Proceedings of the Institution of Mechanical Engineers part o-journal of risk and reliability Article Number: 1748006X20976817 Dec 2020
Collections