Accurate inference of the internal polarising impedance surface and total energy capacity - enabling precise Lithium - Ion battery cell voltage and power prognosis
Tesis
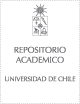
Publication date
2021Metadata
Show full item record
Cómo citar
Orchard Concha, Marcos
Cómo citar
Accurate inference of the internal polarising impedance surface and total energy capacity - enabling precise Lithium - Ion battery cell voltage and power prognosis
Author
Professor Advisor
Abstract
This research focuses on a Hybrid Neural Adaptive State Space Model (NASSM), the purpose of which is to solve the complex problem of accurately characterising the ever changing (non-measurable) polarising impedance multi-dimensional surface and capacity degradation of a Lithium-Ion battery. This is achieved by proposing a novel strategy and architecture to infer these critical battery parameters simultaneously, directly from operational data, avoiding the need of costly off-line testing procedures. The NASSM infers a representational general surface model of the polarising impedance multi-dimensional surface by partially embedding a multi-layer perceptron (or deep neural network), within the hidden state representation and uses Variational Sequential Monte Carlo to infer the parameterisation of said surface as well as the total energy value in order to adapt the model to these changing (degrading) values. Training is performed online with experimental operational data and it is demonstrated that this methodology allows the model to perform accurate predictions of the probability of when a generic battery management system would disconnect the the battery due to the terminal voltage falling below a predefined threshold (a physical constraint). The results are compared to the state of the art on experimental data.
General note
Tesis para optar al grado de Doctor en Ingeniería Eléctrica
Identifier
URI: https://repositorio.uchile.cl/handle/2250/181934
Collections
The following license files are associated with this item: