Improving astronomical time-series classification via data augmentation with generative adversarial networks
Artículo
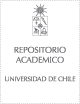
Open/ Download
Access note
Acceso abierto
Publication date
2022Metadata
Show full item record
Cómo citar
García Jara, Germán Eduardo
Cómo citar
Improving astronomical time-series classification via data augmentation with generative adversarial networks
Abstract
Due to the latest advances in technology, telescopes with significant sky coverage will produce millions of astronomical alerts per night that must be classified both rapidly and automatically. Currently, classification consists of supervised machine-learning algorithms whose performance is limited by the number of existing annotations of astronomical objects and their highly imbalanced class distributions. In this work, we propose a data augmentation methodology based on generative adversarial networks (GANs) to generate a variety of synthetic light curves from variable stars. Our novel contributions, consisting of a resampling technique and an evaluation metric, can assess the quality of generative models in unbalanced data sets and identify GAN-overfitting cases that the Fréchet inception distance does not reveal. We applied our proposed model to two data sets taken from the Catalina and Zwicky Transient Facility surveys. The classification accuracy of variable stars is improved significantly when training with synthetic data and testing with real data with respect to the case of using only real data.
Patrocinador
National Agency of Research and Developments Millennium Science Initiative IC12009
National Agency for Research and Development (ANID) grants FONDECYT Regular 1220829
National Agency for Research and Development (ANID) grant: Magister Nacional 2019-22190949
Institute for Applied Computational Science (IACS), Harvard University
Indexation
Artículo de publícación WoS Artículo de publicación SCOPUS
Quote Item
The Astrophysical Journal, 935:23 (14pp), 2022 August 10
Collections
The following license files are associated with this item: