Importance of sample size, data type and prediction method for remote sensing-based estimations of aboveground forest biomass
Artículo
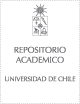
Publication date
2014Metadata
Show full item record
Cómo citar
Fassnacht, F.E.
Cómo citar
Importance of sample size, data type and prediction method for remote sensing-based estimations of aboveground forest biomass
Author
Abstract
Estimates of forest biomass are needed for various technical and scientific applications, ranging fromcarbon and
bioenergy policies to sustainable forest management. As localmeasurements are costly, there is a great interest in
obtaining reliable estimates over large areas from remote sensing data. Currently, such estimates are obtained
with a variety of data sources, statistical methods and prediction standards, and there is no agreement on
what are best practices for this task.
To improve our understanding of how these different methods affect prediction quality, we first conducted a
systematic review of the available literature to identify themost common sensor types and prediction methods.
Based on the review, we identified sample size of the reference points on the ground, prediction method (stepwise
linear regression, support vector machines, randomforest, Gaussian processes and k-nearest neighbor), and
sensor type as themain differences that could potentially affect predictive quality. We then compared those factors
in two case study areas in Germany and Chile, for which airborne discrete return Light Detection And Ranging
(LiDAR) and airborne hyperspectral as well as airborne discrete return LiDAR and spaceborne hyperspectral
data were available. For each factor combination, we calculated Pearson's coefficient of correlation between
observations and predictions (r2) and root mean squared error (RMSE) for bootstrapped estimates using k-fold
cross-validation with a varying number of folds. Finally, Analysis of Variance (ANOVA) was used to quantify
the influence of the factors on the predictive error of the biomass models.
Our results confirm previous findings that predictor data (sensor) type is the most important factor for the accuracy
of biomass estimates, with LiDAR being preferable to hyperspectral data. In contrast to some previous
studies, complementing LiDAR with hyperspectral data did not improve predictive accuracy. Also the prediction
method had a substantial effect on accuracy and was generally more important than the sample size. In most
cases, random forest performed best and stepwise linear models worst, judging from r2 and RMSE under crossvalidation.
Additional results suggested that r2 may deliver unrealistically large values when the hold-out sample
during the cross-validation is too small.
In conclusion, our literature review revealed that different methods for biomass estimation are currently used,
with no general agreement on best practices. In our case studies, we found substantial accuracy differences between
those methods, with LiDAR data, in combination with a random forest algorithm and a large number of
reference sample units on the ground yielding the lowest error for biomass predictions. The comparatively
high importance of the statistical prediction method seems particularly relevant, as they suggest that choosing
the appropriate statistical method may be more effective than obtaining additional field data for obtaining
good biomass estimates. Considering the costs of improving accuracy of global and regional biomass estimates
by ground measurements, it seems sensible to invest in further comparative studies, preferably with a wider
range of sites and including also RADAR sensors, to establish robust best-practice recommendations for obtaining
regional and global biomass estimates from remote-sensing data.
General note
Articulo de publicacion SCOPUS
Patrocinador
German National Space Agency
DLR (Deutsches Zentrum für Luft- und Raumfahrt e.V.)
BioComsa, Consorcio Tecnológico de Biocombustibles S.A., Chile
Identifier
URI: https://repositorio.uchile.cl/handle/2250/120244
DOI: dx.doi.org/10.1016/j.rse.2014.07.028
Quote Item
Remote Sensing of Environment 154 (2014) 102–114
Collections