Discovering geochemical patterns using self-organizing neural networks: a new perspective for sedimentary provenance analysis
Artículo
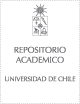
Open/ Download
Publication date
2004-03-01Metadata
Show full item record
Cómo citar
Lacassie Reyes, Juan Pablo
Cómo citar
Discovering geochemical patterns using self-organizing neural networks: a new perspective for sedimentary provenance analysis
Abstract
An unsupervised neural network technique, Growing Cell Structures (GCS), was used to visualize geochemical differences between sandstones of four different sedimentary provenance groups: P1 (mafic), P2 (intermediate), P3 (felsic), and P4 (recycled). Multidimensional data of four sandstone data sets comprising major elements, log-normalized major elements, trace elements, and high field strength elements (HFSE) were projected into colored two-dimensional maps that can be visually and quantitatively interpreted. The cluster structure and variable distributions produced show that each sedimentary provenance group can be distinguished in the neural maps according to a unique combination of major or trace element concentrations. In these terms, the distinguishing features of each provenance group are: P1-high Fe(2)O(3)t, TiO2, MgO, MnO, CaO, P2O5, Sc, V, Cr, and Cu; P2-intermediate Fe(2)O(3)t, TiO2, MgO, MnO, CaO, Sc, V, and Cu; P3-intermediate to high K2O, intermediate SiO2 and Al2O3, low Fe(2)O(3)t and TiO2, and intermediate to low Nb, Rb, and Th; P4-high SiO2, Y, Nb, Rb, Th, Ba, and Zr, coupled with low Al2O3, CaO, Na2O, Fe(2)O(3)t, MgO, MnO, and TiO2. The elemental associations in P1, P2, and P3 reflect petrogenetic evolution of first-cycle sources, whereas the associations in P4 are compatible with the combined effects of recycling, weathering, and heavy mineral concentration.
Quote Item
SEDIMENTARY GEOLOGY 165 (1-2): 175-191 MAR 1 2004
Collections