Toward real-time decentralized reinforcement learning using finite support basis functions
Artículo
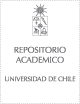
Open/ Download
Access note
Acceso a solo metadatos
Publication date
2017Metadata
Show full item record
Cómo citar
Lobos-Tsunekawa, Kenzo
Cómo citar
Toward real-time decentralized reinforcement learning using finite support basis functions
Abstract
This paper addresses the design and implementation of complex Reinforcement Learning (RL) behaviors where multi-dimensional action spaces are involved, as well as the need to execute the behaviors in real-time using robotic platforms with limited computational resources and training times. For this purpose, we propose the use of decentralized RL, in combination with finite support basis functions as alternatives to Gaussian RBF, in order to alleviate the effects of the curse of dimensionality on the action and state spaces respectively, and to reduce the computation time. As testbed, a RL based controller for the in-walk kick in NAO robots, a challenging and critical problem for soccer robotics, is used. The reported experiments show empirically that our solution saves up to 99.94% of execution time and 98.82% of memory consumption during execution, without diminishing performance compared to classical approaches.
Indexation
Artículo de publicación SCOPUS
Identifier
URI: https://repositorio.uchile.cl/handle/2250/169506
DOI: 10.1007/978-3-030-00308-1_8
ISSN: 16113349
03029743
Quote Item
Lecture Notes in Computer Science (including subseries Lecture Notes in Artificial Intelligence and Lecture Notes in Bioinformatics), Volumen 11175 LNAI, 2017
Collections