Informational content of cosine and other similarities calculated from high-dimensional Conceptual Property Norm data
Artículo
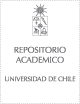
Open/ Download
Access note
Acceso Abierto
Publication date
2020Metadata
Show full item record
Cómo citar
Canessa, Enrique
Cómo citar
Informational content of cosine and other similarities calculated from high-dimensional Conceptual Property Norm data
Abstract
To study concepts that are coded in language, researchers often collect lists of conceptual properties produced by human subjects. From these data, different measures can be computed. In particular, inter-concept similarity is an important variable used in experimental studies. Among possible similarity measures, the cosine of conceptual property frequency vectors seems to be a de facto standard. However, there is a lack of comparative studies that test the merit of different similarity measures when computed from property frequency data. The current work compares four different similarity measures (cosine, correlation, Euclidean and Chebyshev) and five different types of data structures. To that end, we compared the informational content (i.e., entropy) delivered by each of those 4 x 5 = 20 combinations, and used a clustering procedure as a concrete example of how informational content affects statistical analyses. Our results lead us to conclude that similarity measures computed from lower-dimensional data fare better than those calculated from higher-dimensional data, and suggest that researchers should be more aware of data sparseness and dimensionality, and their consequences for statistical analyses.
Patrocinador
Comision Nacional de Investigacion Cientifica y Tecnologica (CONICYT)
CONICYT FONDECYT
1200139
Indexation
Artículo de publicación ISI Artículo de publicación SCOPUS
Quote Item
Cognitive Processing Jul 2020
Collections
The following license files are associated with this item: