Screening of COVID-19 cases through a Bayesian network symptoms model and psychophysical olfactory test
Artículo
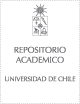
Open/ Download
Access note
Acceso abierto
Publication date
2021Metadata
Show full item record
Cómo citar
Eyheramendy, Susana
Cómo citar
Screening of COVID-19 cases through a Bayesian network symptoms model and psychophysical olfactory test
Author
Abstract
The sudden loss of smell is among the earliest and most prevalent symptoms of
COVID-19 when measured with a clinical psychophysical test. Research has shown
the potential impact of frequent screening for olfactory dysfunction, but existing
tests are expensive and time consuming. We developed a low-cost ($0.50/test)
rapid psychophysical olfactory test (KOR) for frequent testing and a model-based
COVID-19 screening framework using a Bayes Network symptoms model. We
trained and validated the model on two samples: suspected COVID-19 cases in
five healthcare centers (n = 926; 33% prevalence, 309 RT-PCR confirmed) and
healthy miners (n = 1,365; 1.1% prevalence, 15 RT-PCR confirmed). The model
predicted COVID-19 status with 76% and 96% accuracy in the healthcare and
miners samples, respectively (healthcare: AUC = 0.79 [0.75–0.82], sensitivity:
59%, specificity: 87%; miners: AUC = 0.71 [0.63–0.79], sensitivity: 40%, specificity: 97%, at 0.50 infection probability threshold). Our results highlight the potential for low-cost, frequent, accessible, routine COVID-19 testing to support
society’s reopening.
Patrocinador
Technological Adoption Fund SiEmpre from SOFOFA Hub (CORFO)
ANID through the Millennium Science Initiative Program ICN17 002
ANID Millennium Science Initiative Program NCN17 081
ANID/FONDAP CIGIDEN 15110017
ANID FONDECYT 1200146
ANID FONDECYT de Iniciacion 11190871
Indexation
Artículo de publícación WoS
Quote Item
iScience 24, 103419, December 17, 2021
Collections
The following license files are associated with this item: