Multi-feature computational framework for combined signatures of dementia in underrepresented settings
Artículo
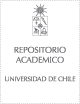
Open/ Download
Access note
Acceso abierto
Publication date
2022Metadata
Show full item record
Cómo citar
Moguilner, Sebastian
Cómo citar
Multi-feature computational framework for combined signatures of dementia in underrepresented settings
Author
- Moguilner, Sebastian;
- Birba, Agustina;
- Fittipaldi, Sol;
- González Campos, Cecilia;
- Tagliazucchi, Enzo Rodolfo;
- Reyes, Pablo;
- Matallana, Diana;
- Parra, Mario A.;
- Slachevsky Chonchol, Andrea María;
- Farías Gontupil, Gonzalo Andrés;
- Cruzat Grand, Josefina;
- García, Adolfo;
- Eyre, Harris A.;
- La Joie, Renaud;
- Rabinovici, Gil;
- Whelan, Robert;
- Ibáñez, Agustín;
Abstract
Objective. The differential diagnosis of behavioral variant frontotemporal dementia (bvFTD) and
Alzheimer’s disease (AD) remains challenging in underrepresented, underdiagnosed groups,
including Latinos, as advanced biomarkers are rarely available. Recent guidelines for the study of
dementia highlight the critical role of biomarkers. Thus, novel cost-effective complementary
approaches are required in clinical settings. Approach. We developed a novel framework based on a
gradient boosting machine learning classifier, tuned by Bayesian optimization, on a multi-feature
multimodal approach (combining demographic, neuropsychological, magnetic resonance imaging
(MRI), and electroencephalography/functional MRI connectivity data) to characterize
neurodegeneration using site harmonization and sequential feature selection. We assessed 54
bvFTD and 76 AD patients and 152 healthy controls (HCs) from a Latin American consortium
(ReDLat). Main results. The multimodal model yielded high area under the curve classification
values (bvFTD patients vs HCs: 0.93 (±0.01); AD patients vs HCs: 0.95 (±0.01); bvFTD vs AD
patients: 0.92 (±0.01)). The feature selection approach successfully filtered non-informative
multimodal markers (from thousands to dozens). Results. Proved robust against multimodal
heterogeneity, sociodemographic variability, and missing data. Significance. The model accurately
identified dementia subtypes using measures readily available in underrepresented settings, with a
similar performance than advanced biomarkers. This approach, if confirmed and replicated, may
potentially complement clinical assessments in developing countries.
Patrocinador
Consejo Nacional de Investigaciones Cientificas y Tecnicas (CONICET)
ANID/FONECYT Regular 1170010
ANPCyT
FONCyT 2017-1820
ANID/FONDAP 15150012
Takeda Pharmaceutical Company Ltd CW2680521
Sistema General de Regalias BPIN2018000100059
Universidad del Valle CI 5316
Alzheimer's Association UK-20-639295
MULTI-PARTNER CONSORTIUM TO EXPAND DEMENTIA RESEARCH IN LATIN AMERICA
National Institutes of Health, National Institutes of Aging R01 AG057234
Alzheimer's Association SG-20-725707
Indexation
Artículo de publícación WoS Artículo de publicación SCOPUS
Quote Item
J. Neural Eng. 19 (2022) 046048
Collections
The following license files are associated with this item: