Total mutational load and clinical features as predictors of the metastatic status in lung adenocarcinoma and squamous cell carcinoma patients
Artículo
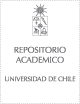
Open/ Download
Access note
Acceso abierto
Publication date
2022Metadata
Show full item record
Cómo citar
Oróstica Tapia, Karen Yasmine
Cómo citar
Total mutational load and clinical features as predictors of the metastatic status in lung adenocarcinoma and squamous cell carcinoma patients
Author
Abstract
Background: Recently, extensive cancer genomic studies have revealed mutational and clinical data of large cohorts of cancer patients. For example, the Pan‑Lung Cancer 2016 dataset (part of The Cancer Genome Atlas project), sum‑ marises the mutational and clinical profiles of different subtypes of Lung Cancer (LC). Mutational and clinical signa‑ tures have been used independently for tumour typification and prediction of metastasis in LC patients. Is it then possible to achieve better typifications and predictions when combining both data streams? Methods: In a cohort of 1144 Lung Adenocarcinoma (LUAD) and Lung Squamous Cell Carcinoma (LSCC) patients, we studied the number of missense mutations (hereafter, the Total Mutational Load TML) and distribution of clinical variables, for different classes of patients. Using the TML and different sets of clinical variables (tumour stage, age, sex, smoking status, and packs of cigarettes smoked per year), we built Random Forest classification models that calculate the likelihood of developing metastasis. Results: We found that LC patients different in age, smoking status, and tumour type had significantly different mean TMLs. Although TML was an informative feature, its effect was secondary to the "tumour stage" feature. However, its contribution to the classification is not redundant with the latter; models trained using both TML and tumour stage performed better than models trained using only one of these variables. We found that models trained in the entire dataset (i.e., without using dimensionality reduction techniques) and without resampling achieved the highest perfor‑ mance, with an F1 score of 0.64 (95%CrI [0.62, 0.66]). Conclusions: Clinical variables and TML should be considered together when assessing the likelihood of LC patients progressing to metastatic states, as the information these encode is not redundant. Altogether, we provide new evi‑ dence of the need for comprehensive diagnostic tools for metastasis.
Patrocinador
Gobierno de Chile Programa Centro Internacional de Excelencia CORFO 13CEE2-21602
Comisión Nacional de Investigación Científica y Tecnológica (CONICYT)
CONICYT PIA/ANILLOS ANID ACT210079
Becas CONICYT PIA FB0001
Centro de Biotecnología y Bioingeniería
Beca de doctorado de KYO 21182123
Indexation
Artículo de publícación WoS Artículo de publicación SCOPUS
Quote Item
Journal of Translational Medicine (2022) 20:373
Collections
The following license files are associated with this item: