Forecasting gold price changes: Rolling and recursive neural network models
Artículo
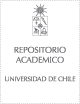
Open/ Download
Publication date
2008Metadata
Show full item record
Cómo citar
Parisi Fernández, Antonino
Cómo citar
Forecasting gold price changes: Rolling and recursive neural network models
Abstract
This paper analyzes recursive and rolling neural network models to forecast one-step-ahead sign variations in gold price. Different combinations of techniques and sample sizes are studied for feed forward and ward neural networks. The results shows the rolling ward networks exceed the recursive ward networks and feed forward networks in forecasting gold price sign variation. The results support the use of neural networks with a dynamic framework to forecast the gold price sign variations, recalculating the weights of the network on a period-by-period basis, through a rolling process. Our results are validated using the block bootstrap methodology with an average sign prediction of 60.68% with a standard deviation of 2.82% for the rolling ward net. © 2008 Elsevier B.V. All rights reserved.
Indexation
Artículo de publicación SCOPUS
Identifier
URI: https://repositorio.uchile.cl/handle/2250/154683
DOI: 10.1016/j.mulfin.2007.12.002
ISSN: 1042444X
Quote Item
Journal of Multinational Financial Management, Volumen 18, Issue 5, 2018, Pages 477-487
Collections