An Interactive Framework for Learning Continuous Actions Policies Based on Corrective Feedback
Artículo
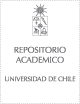
Open/ Download
Publication date
2019Metadata
Show full item record
Cómo citar
Celemin, Carlos
Cómo citar
An Interactive Framework for Learning Continuous Actions Policies Based on Corrective Feedback
Author
Abstract
© 2018, Springer Science+Business Media B.V., part of Springer Nature.The main goal of this article is to present COACH (COrrective Advice Communicated by Humans), a new learning framework that allows non-expert humans to advise an agent while it interacts with the environment in continuous action problems. The human feedback is given in the action domain as binary corrective signals (increase/decrease the current action magnitude), and COACH is able to adjust the amount of correction that a given action receives adaptively, taking state-dependent past feedback into consideration. COACH also manages the credit assignment problem that normally arises when actions in continuous time receive delayed corrections. The proposed framework is characterized and validated extensively using four well-known learning problems. The experimental analysis includes comparisons with other interactive learning frameworks, with classical reinforcement learning approaches, and with human teleoperators tryi
Indexation
Artículo de publicación SCOPUS
Identifier
URI: https://repositorio.uchile.cl/handle/2250/171299
DOI: 10.1007/s10846-018-0839-z
ISSN: 15730409
09210296
Quote Item
Journal of Intelligent and Robotic Systems: Theory and Applications, Volumen 95, Issue 1, 2019, Pages 77-97
Collections