A fast hybrid reinforcement learning framework with human corrective feedback
Artículo
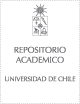
Publication date
2019Metadata
Show full item record
Cómo citar
Celemin, Carlos
Cómo citar
A fast hybrid reinforcement learning framework with human corrective feedback
Author
Abstract
Reinforcement Learning agents can be supported by feedback from human teachers in the learning loop that guides the learning process. In this work we propose two hybrid strategies of Policy Search Reinforcement Learning and Interactive Machine Learning that benefit from both sources of information, the cost function and the human corrective feedback, for accelerating the convergence and improving the final performance of the learning process. Experiments with simulated and real systems of balancing tasks and a 3 DoF robot arm validate the advantages of the proposed learning strategies: (i) they speed up the convergence of the learning process between 3 and 30 times, saving considerable time during the agent adaptation, and (ii) they allow including non-expert feedback because they have low sensibility to erroneous human advice.
Indexation
Artículo de publicación SCOPUS
Identifier
URI: https://repositorio.uchile.cl/handle/2250/171513
DOI: 10.1007/s10514-018-9786-6
ISSN: 15737527
09295593
Quote Item
Autonomous Robots (2019) 43:1173–1186
Collections